PhD thesis of Félix Raimundo
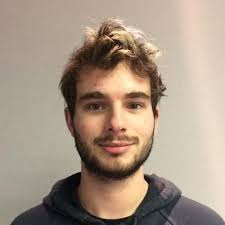
The emergence of resistance to chemotherapy and targeted therapies is a major challenge for the treatment of cancer. Genetic heterogeneity within untreated tumors is now considered to be a key determinant of resistance; sub-population of cells bearing a mutation conveying resistance can survive and be selected in a Darwinian process. In addition, non-genetic and particularly transcriptional and epigenetic mechanisms are anticipated to play a role in the adaptation of cancer cells confronted with environmental, metabolic or therapy-related stresses. Modulation of chromatin structure via histone modification is a major epigenetic mechanism and key regulator of gene expression, however, the contribution of chromatin heterogeneity to tumor evolution remains unknown, mostly due to the lack of methods to study it in tumors. The Vallot lab, in collaboration with ESPCI, has now developed and validated a droplet microfluidics workflow for single-cell chromatin immunoprecipitation sequencing (scChIP-seq), with dedicated analytical tools, to analyze the epigenome at single-cell resolution of thousands of cells with a coverage of more than 10,000 loci/cell. Thanks to a first set of analyses, based on linear models, we have studied the heterogeneity of chromatin states in breast tumor samples, resistant or not to chemotherapy. Our preliminary analyses revealed a rare subgroup of cells within a sensitive tumor that harbor epigenetic traits similar to the ones of cells from the resistant tumor (Grosselin et al, under evaluation). Such observation could suggest that a resistant epi-clone' pre-exist in the original tumor prior to treatment. The analysis and further modeling of the obtained datasets are challenging and need thorough statistical insight and mathematical modeling to extract relevant information. Among others challenges, these datasets are of high dimensions (more than 50 000 loci in over 10 000 cells), zero-inflated and contain a high number of missing values and false negative data points. We now wish to take advantage of deep learning methods to extract the most relevant features and groups from these unique single-cell omics datasets, characterizing for the first time the epigenome of cancer cells at single cell resolution. Teaming up with Jean-Philippe Vert at the Google Lab, we will use unsupervised learning, to develop algorithms to perform (i) imputation of missing values to circumvent the technological limitations of single-cell epigenomics, (ii) features extraction and dimensionality reduction, (iii) group characterization (ie find features specific of each groups) and (iv) pseudo-time reconstruction. In contrast to supervised deep learning methods, with data labels and training sets, we wish here to dig into the structure of the learning algorithms (hidden layers and nodes) to extract information and interpret these components at the biological level. Our final aim is to characterize the heterogeneity of chromatin states in breast cancer, naturally occurring or in response to chemotherapy.