Master thesis of Bastien Batardière
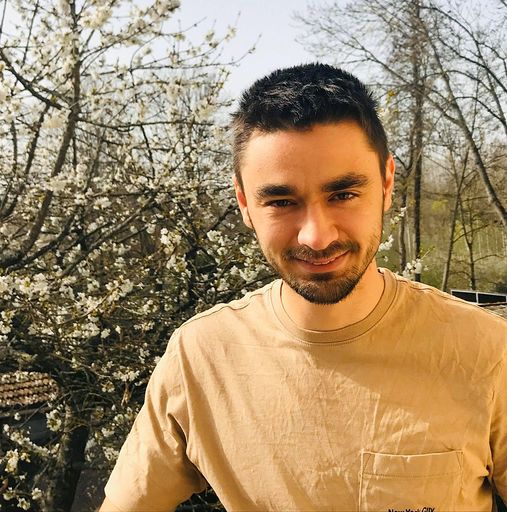
The Poisson lognormal model is a multivariate latent variable model that provides a general framework for modeling covariations between variables (genes, species) taking into account possible environmental effects in the case of count data. We have proposed various variants of this model (parsimonious covariance structure, diagonal, spherical, rank constraint, mixture model) and applications to the multivariate analysis of count tables (network inference, probabilistic PCA, discriminant analysis) see here.
Within the framework of the PLN model, and motivated by the characteristics of single cell data, Bastien’s internship aims to explore various research questions:
-
Inference strategy - alternative to variational estimation: non-approximate log-likelihood estimation (gradient estimation by stochastic approach).
-
Optimization - study of variants of the stochastic gradient descent for the optimization of various criteria (ELBO or log-likelihood): Momentum, Nesterov, AdaGrad, RMSProp, Adam, variance reduction.
-
Statistical inference - study of the convergence of variational estimators, consistency