PhD thesis of Elias Ventre
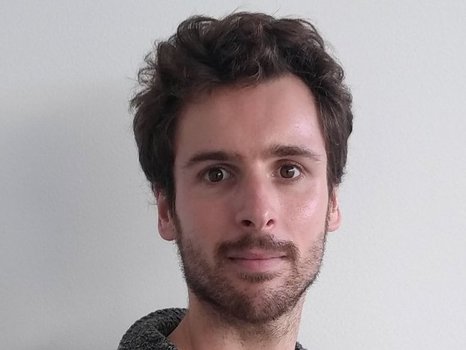
Differentiation is the process whereby a cell acquires a specific phenotype, by differential gene expression as a function of time. We can represent this process as a trajectory in the gene expression space. A popular vision of this evolution, introduced by Waddington, is to compare cells to marbles following probabilistic trajectories, as they roll through a developmental landscape of ridges and valleys. The landscape is often regarded to be shaped by the underlying dynamics of a Gene Regulatory Network.
Single cell technologies have shown that differentiation is a highly stochastic process. A model of gene expression able to recreate the noisy nature of gene expression from the GRN was recently introduced in our team. This mechanistic model, based on a description of the chemical reactions between promoters, ARN and proteins into the nucleus, defines a Piecewise Deterministic Markov Processes (PDMP) for each gene. Its results correspond accurately to the non-Gaussian distribution of the single cell gene expression data. A network of such PDMP allows to obtain a system of Partial Differential Equation describing the evolution of the distribution of a cell in the gene expression space.
In a first part, we will develop a mathematical framework and a method to express the energetic landscape of cell differentiation processes when described by the PDMP model. The study of landscape deformation is a powerful tool to go further into the concept of metastability, which seems inherent to cell differentiation processes as a consequence of the limited number of existing phenotype. This suggests that our model can be reduced to a simple discrete process on a small number of cell types : we will see how to derive rigorously this process from the mechanistic model.
In a second part, we will use this reduction to take a broader perspective, developing a multiscale approach to study other sources of cellular variability, including but not limited to, cell division.